The benefits of embracing technology are attractive, and organizations are rapidly adopting the best practices of this digital-first climate. Businesses can emerge competitive and make sounder decisions with the help of rich insights the modern tools offer. Hence, the attention of decision-makers on modernizing their business processes is more than ever. In terms of data management, data migration is the first step in digital transformation. However, big data migration demands time, budget, and resources. With a familiarity with the big data migration mistakes to avoid, businesses can reap a higher return on investment in data migration.
What is data migration?
Data migration involves moving data from one place to another and retiring legacy systems to embrace new data applications. The need for big data migration arises when an organization intends to move its data assets to the cloud from the existing on-premise data setup.
Furthermore, several reasons given here might trigger the necessity of data migration:
- Database upgradation
- Introducing a new data warehouse
- Replacing servers or storage devices
- Consolidating or decommissioning data center
- Reconstructing the enterprise data system
- Technology implementation into the existing systems
- To merge new data during an acquisition
Irrespective of the reason, data migration is the first step in the process of migrating on-premises IT infrastructure to a cloud computing setup. If done right, data migration can lay the foundation for effective data systems that aid business growth.
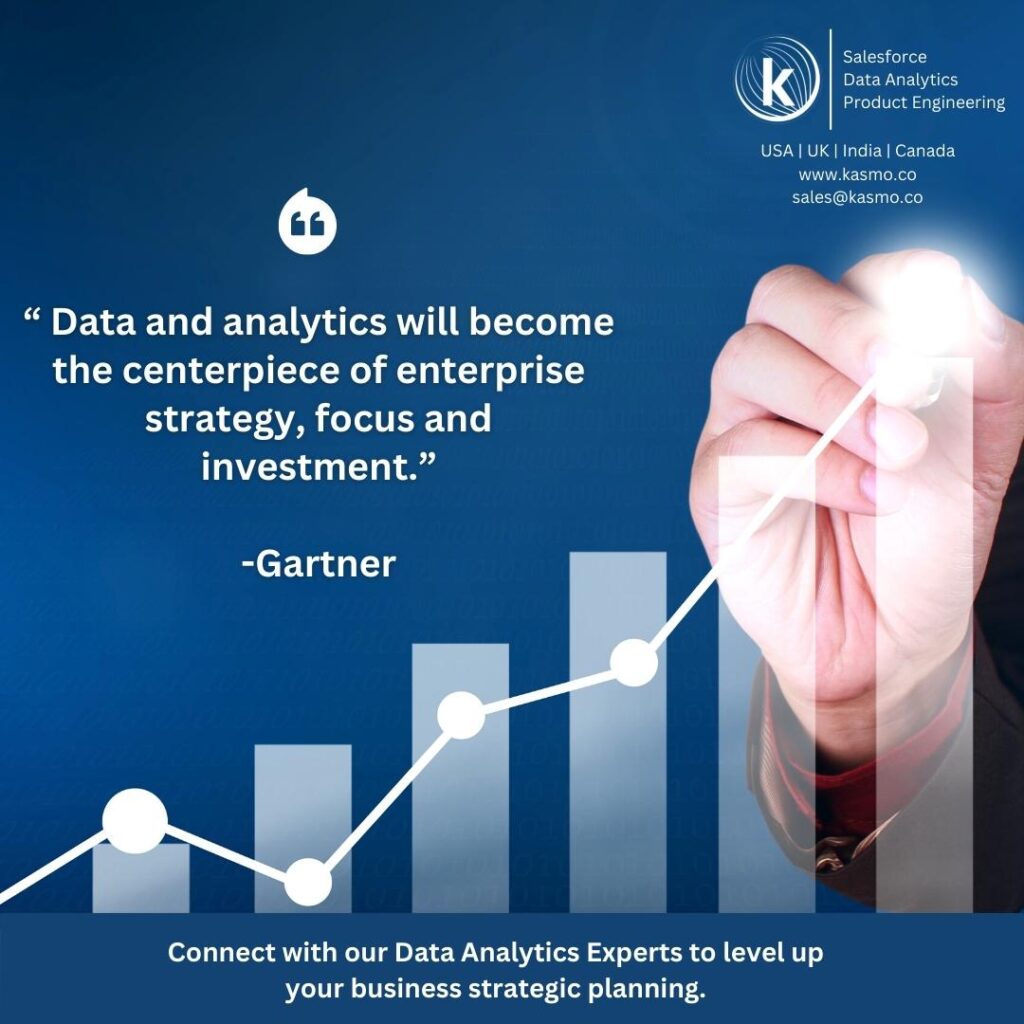
Data Migration Types
Businesses may opt to migrate data to cloud or another storage appliance offline based on their cost and performance requirements. Accordingly, data migration falls into two broad categories:
- Online migration: Here data is migrated across the Internet or a private wireless connection.
- Offline migration: Here data is migrated through a storage application which is physically shipped between the origin and the target location.
The choice between these two types of data migration depends on the data volume, speed, workloads involved and security preferences.
A data migration strategy is essential to reap the benefits of data migration and prevent big data migration mistakes that may often derail projects.
Data migration steps
A well-rounded ETL (Extract, Transform, and Loading) data migration includes the following four critical phases:
- Pre-migration phase
- Migration phase
- Post-migration
- Data Synchronization
Pre-migration phase:
This stage is also called the planning phase and includes analysis and planning for the data migration as follows:
- Evaluation of source data and its fitness for the target system.
- Data profiling to establish patterns and relationships for better data consolidation.
- Selecting the best data migration solution.
- Budget planning, resource pooling, and timeline estimation.
- Estimating the data security measures and arranging for the necessary tools.
- Planning for data backup as an additional measure in case of unforeseen outcomes.
Migration phase:
Migration is the second stage which comprises three main sub-phases: Data Extraction, Data Transformation, and Data Validation.
- At this stage, the Extraction and Loading of data take place.
- Ensuring business continuity throughout the extraction and loading.
- Monitoring the process for accuracy and auditing the transfer process for guidelines.
- Regular testing is necessary to check if loading takes place as intended and data works without issues.
Post-migration:
Once the data loads into the destination, it is time to check its accuracy and expected functionality. Performance deviations indicate the necessity for further probing why and where the process has gone awry. Closing the post-migration process without checking for discrepancies can be one of the data migration mistakes.
Data synchronization and monitoring
Synchronization ensures that migrated data works effectively at the destination without any hiccups. Successful synchronization indicates that the target system is fully functional.
At each stage, choose the right tool to plan, check, audit, and implement. Big data migration mistakes at any stage can prove unfruitful for the investment, time, and effort put in.
“We’re entering a new world in which data may be more important than software.” – Tim O’Reilly, founder, O’Reilly Media.
Here is the guide that brings the list of 10 data migration mistakes that arise and explains why it is crucial to prevent them.
1. Not preparing the source data
While initiating data migration, it is vital to clean the source data. Define what comprises your source data and look for repetitions, duplicates, spelling errors, and gaps in the source data. This simplifies the data configuration with new assets and eliminates the data reprocessing cost. At this step, cleanse the source data to make it fit the post-migration categories.
2. Neglecting Data Discovery
Data is the new fuel for organizations, and data discovery is what unravels the wealth hidden in the data. Organizations might not dedicate sufficient resources to data discovery. Reasons vary from the inability to cope with the data volume and information velocity to considering the investment in data discovery as an additional expense.
On the contrary, allocating enough time for data discovery can help reveal actionable insights that help business decision-making. Data discovery transforms untidy, unorganized data into structured, rich data that guide toward KPIs, patterns, and circulations.
3. Fragmented Data Movement Strategy
In the absence of a central data repository, data arriving from disparate sources gets stored across different data management systems. Data fragmentation can hamper the data access to the team.
When planning for data migration, it is vital to account for such fragmented data. Discounting the importance of fragmented data can impact system and operational efficiency.
In other words, fragmented data movement is one of the most common data migration mistakes that lead to data redundancy and the consumption of additional data storage resources. This also leaves the organizations in the dark about the whereabouts of the data origin, access, and usage, creating serious compliance or security risks and storage optimization concerns.
4. Skipping Data Audit and Validation
Data audit allows organizations to discover and eliminate system insufficiencies. It is a critical step to control data migration costs and time. A well-planned data audit gives control of data by removing unnecessary data storage and fixing the deficiencies in the data infrastructure.
Another crucial step that follows a data audit is data validation. It includes ensuring the quality of migrated data and eliminating the scope for business disruption once the data goes live after migration.
Depending on the availability of resources, data volume, and migration strategy, data validation may happen in a single stage or multiple phases.
5. Collaboration silos
One of the data migration mistakes is the inability to comprehend the value of collaboration in driving data migration. Invite all stakeholders to participate in data migration to allow seamless data access, security, and ownership post-migration.
Collaboration in data migration leads to a better understanding of data migration drivers, faster addressing of data migration issues, and provides a comprehensive view of the end-to-end data traversal.
6. Outdated data migration tools
The selection of data migration tools impacts the efficiency and timeliness of data migration. Using outdated tools that offer limited scalability to save costs and proceeding with data migration without expert help are data migration mistakes to avoid.
Consider the organization’s data migration needs and goals, training required, cost, reliability, existing tools, data volume, sources, and security to select the best data migration tool.
7. Poor Data Quality
While moving data from legacy systems to the latest applications, not maintaining data quality is one of the data migration mistakes. Poor quality data, if migrated without enhancement, can cause issues ranging from the subpar performance of the data systems, failure to achieve the set data migration goals, poor business intelligence, and disruption in business processes.
Businesses might also face the challenges of credibility loss, compliance issues, system integration issues, customer dissatisfaction due to erroneous data, and additional cost to rectify the related data quality issues.
The term data quality can differ ranging from data volume and type. Below are ways to enhance data quality irrespective of the data type:
- eliminate duplicate records
- correct the data formats
- maintain hierarchies and relationships between data models
- apply data scrubbing/cleansing methods.
8. Incohesive Business Operation Planning
Data migration may sound like a straightforward process – moving data from old systems to newer ones. The process involves intense operational and technical planning, including backup creation, testing, result validation, and more.
Data migration is successful only when legacy systems can be shut down, and the new ones are fully functional. Operational planning is vital to ensure business continuity throughout this process.
9. Lack of documented process for data migration
A documented process serves as a blueprint for data migration. It guides all critical stages of the task and ensures that all stakeholders are informed of the practices. A documented strategy clarifies the point of contact for each stage, the hierarchy for the follow-up, and the deliverables from each stakeholder involved in the data migration process.
10. Security and Compliance
Automated compliance must be an essential part of any data migration strategy. Failure to include compliance can be one of the data migration mistakes that could impact customer trust and the business reputation.
Additionally, implementing the best data security measures throughout the data migration is crucial. Businesses must ensure that the data migration partner adheres to all security and compliance guidelines.
Approach us for all your data migration needs
Data migration is often an underestimated business transformation approach. Given the upkeep the process demands and the benefits it imparts, always consider expert help for your business data migration.
In the competitive digital climate, business success depends on selecting optimal environment and extracting the value of data to the fullest. Data migration needs thorough understanding of your business needs and the end goals.
The planning, strategy and implementation can decide the success of data migration from its earliest stages. Ask our experts how to simplify your data migration process within your timelines and desired outcomes. Email us at sales@kasmo.co or send us a message right now for a free consultation.
Read Next: Top 10 Data Analytics Trends in 2023